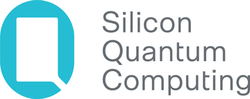
PhD Scholarship in Quantum Machine Learning
Silicon Quantum Computing
Posted 12 days ago
Quantum-enhanced machine learning with semiconductor quantum dots
Join a world-class team at the cutting edge of technology, focussed on making quantum computers a reality
Collaborate with a diverse, multicultural team across the full stack quantum computer
An exciting opportunity exists for a PhD candidate to undertake a unique academic-industry program involving a collaboration between Silicon Quantum Computing Pty Ltd (SQC), the global leader in the manufacture and measurement of quantum processors engineered with atomic precision, UNSW Sydney, and CSIRO.
This prestigious Next Generation PhD scholarship stands out as it includes 11 weeks of funded coursework focusing on a holistic approach to problem-solving with technology. Diverse topics include deep learning, computer vision, ethics, decision making under uncertainty, innovation, and entrepreneurship.
Project Summary
Analogue quantum simulation is an emerging field for quantum chemistry, materials design, and for solving optimisation problems. The use of analogue rather than digital quantum systems allows for the direct mapping of high-valued computational problems onto near-term hardware that may offer an advantage over classical methods. Semiconductor quantum dots have recently been shown to exhibit the controllability and precision required to for analogue quantum simulation of complex physical systems such as magnetism and topological phases in condensed matter. At Silicon Quantum Computing (SQC), we are building a scalable platform for both digital and analogue quantum computation. The digital architecture relies on the spins of single electrons and phosphorus nuclei embedded in a silicon crystal. However, the analogue approach focuses on the use of quantum dots formed using the same phosphorus donors in silicon to directly simulate “atoms” in one- and two-dimensional lattices. Using these quantum processors, we can embed computationally difficult problems to be used as a feature generator for machine learning.
This project will focus on the design, theoretical understanding and measurement of analogue quantum feature generators for quantum-enhanced machine learning. The analogue processors will be fabricated with sub-nanometre precision using a UHV scanning tunnelling microscopy hydrogen-lithography combined with phosphine dosing. The fabricated processors will then be measured in a He3 dilution refrigerator operating at a few mK using various electrical measurement techniques. The successful student will be privy to the entire fabrication and measurement process and can work with internal/external collaborators in condensed matter and quantum chemistry problems. Depending on the processor different analysis/control techniques may be developed including machine learning, feedback controls, and radio-frequency measurement techniques.
This position reports to Dr Sam Gorman and works alongside the full stack team (staff and students) funded by SQC.
Candidate experience
Candidates for this project should have a background in quantum computing, machine learning, or condensed matter physics. Experience and knowledge of the theory of machine learning and/or statistics is highly desirable. General research experience and knowledge of cryogenic systems are also highly desirable with a significant component of the PhD focused on measurements of cryogenic systems.
About SQC
SQC is an Australian research and development company, with the specific aim of building a quantum computer based on atom qubits in silicon.
SQC is a well-funded Australian company formed by the Commonwealth Government, the New South Wales State Government, Commonwealth Bank of Australia, Telstra, and UNSW Sydney.
SQC is seeking to commercialise silicon quantum computing technology developed in Australia – technology that has the potential to have a global impact.
SQC’s work is building on more than 20 years of world-leading research by the Centre of Excellence which includes the development of dedicated manufacturing and measurement techniques for an atom-based quantum computer in silicon.
This role is located at SQC on UNSW Sydney campus.
Eligibility
To be eligible for a scholarship, applicants must be domestic students as per the Higher Education Support Act at the time of award. Domestic students include:
Australian citizens,
Australian permanent residents,
a person entitled to stay in Australia, or to enter and stay in Australia, without any limitation as to time, or
a New Zealand citizen.
As determined by the Faculty Higher Degree Committee (HDC), the minimum requirement for admission to a PhD at UNSW is:
a Bachelor degree with first or upper second-class Honours, or
a completed Master by Research with a substantial research component and demonstrated capacity for timely completion of a high-quality research thesis.
The PhD application process is available here: https://www.unsw.edu.au/research/hdr/application
Key Requirements
Students must register and complete the coursework component of the Next Generation Graduates Program within the first 18 months of receipt of a scholarship.
Students who receive a scholarship are expected to undertake their training in Australia and endeavour to remain in Australia for two years following completion of their degree.
Remuneration
Stipend rate (p.a.): $41,650
Training (p.a.): $5,000
Travel (total): $5,000
Thesis allowance (total): $840
Contact
About Silicon Quantum Computing
This company does not have any further information provided at this time. We encourage you to research the company by searching for them to learn more about the company or role in question before applying.
PhD Scholarship in Quantum Chemistry Simulation
Silicon Quantum Computing
PhD/Honours Scholarships in VR/AR - AI fusion
University of Tasmania
PhD Scholarship: Data-driven spatio-temporal sewer pipe network assessment
Griffith University
CSIRO Next Gen Scholarships - Quantum IT: Industry Readiness and Applications
Monash University
Slitting Machine Operator
Boomerang Tapes
Cancer Epidemiology, Machine Learning & Data Sharing
Scholarships